The explosion in data and the thirst for more and more data continues and shows no signs of abating. However, despite the noise the conversation has barely moved forward in 20 years, it is still focused on more data and not quality, which is surprising.
Yes, the more data you have, the better predictive models you can build. But only if the data is valid, accurate and a good representation of the future. In other words, it's good quality data we want, not just a sheer breadth of data. Only good quality data generates useful predictive models and reports, that enable firms to reach that golden objective of “profitable growth”.
Data quality is a term that gets banded around like confetti these days. Now there is a mini-industry surrounding data quality all under the data governance banner. Don’t get me wrong, I am a firm supporter of data governance, after all, that drives good data, however, I do not think that it has moved the data quality agenda forward or the overall data conversation.
What is data quality?
Firstly, it is about accuracy and then we consider completeness, consistency, timeliness, validity and formatting. That is pretty much, in my view, the basics of data quality.
To demonstrate good quality data, firms typically launch a project to inventory their data assets (data schemas and data dictionaries) and assign data stewards (from the business) who are tasked with owning and defining a set of business rules. So far so good... But, for those who worked as actuaries/ statisticians 20 years ago (like me!), that’s not new! It’s just that these items were done usually by the analyst (me again!!) working with the data. One of my first projects as a fresh graduate was to support the development of the motor pricing database that was developed by the pricing team (really this crazy, but quite lovable Quebecer), which I used to build all the models for price optimisation.
So, really all that has happened in the last 20-odd years is that we’ve shifted the burden of data quality onto data engineers, set up a layer of management, and given responsibility to business users (the actuary/statistician) for data integrity. Realistically the data quality conversation has not moved on, it’s just shifted across resources. I am surprised that the conversation about data quality has not focused on data preparation. Which is where the ‘real money’ is.
Don’t underestimate the value of Data Preparation
This is such a key activity that is often overlooked. Typically, it is left up to the business user of the data to complete data preparation for its intended purpose. And while this is a pragmatic approach, that is not really driving the data quality agenda forward. There is a gulf of difference in value generated as the quality of the data improves ..
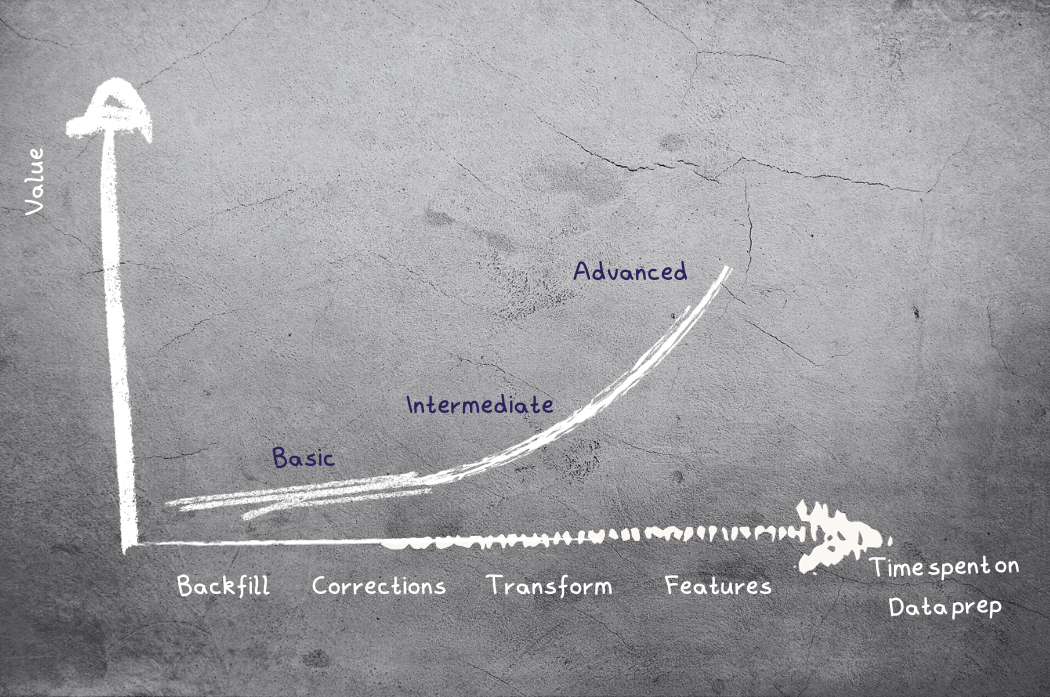
If your data is not prepared adequately, then there is a real risk that the data used is not representative of the future. Thus any model (or even report) will indicate misleading decisions and result in poor business performance. Worse the business will struggle to understand why and fall back to standard explanations such as “it’s a challenging market”.
For me, data prep is so much more than doing basic data cleansing and a bit of supporting exploratory data analysis (EDA). It’s really about understanding is this data a good representation of the future, applying transformations and create new features to represent the current environment. I am also usually, brutal with what data to exclude, it leads to much simpler, cleaner, and usually more predictive models that tend to be quite consistent over time.
So, spend time on data preparation so you can produce better models that, and often much better actuals vs. expected results after implementing to production.
Don’t underestimate the value of data preparation, it should be the cornerstone of any data project.